Join us for this fresh episode as Receivables Roundtable Founder, Adam Parks talks with Jeffrey Shaffer, VP, Information Technology & Analytics at Unifund CCR about prediction models, disparate impact analysis, the future of generative AI, and more!
Listen to Your Favorite Podcasts
About Company
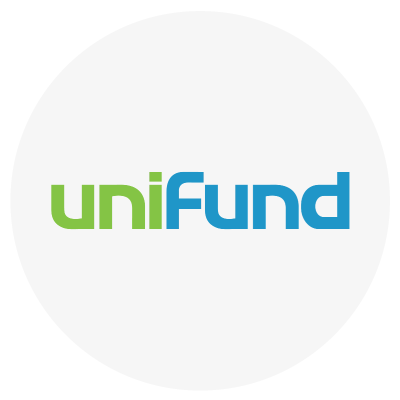
Unifund exists at the intersection of performance and compliance. We are an accounts receivable portfolio investment firm that specializes in using technology, analytics, and machine learning to purchase, manage, service, and liquidate distressed consumer receivables.
About The Guest
Transcript
Adam:
Hello, everybody. Adam Parks here with another episode of receivables roundtable today. I'm here with a recurring guest. I'm going to call him a guest professor here for us teaching us about analytics, data mining, data analytics and artificial intelligence, machine learning, large language models. I mean, this man really has a lot of information to share with us today. So without too much to do, I have Mr. Jeffrey Schaefer, who is the VP of IT and analytics with Unifund. How you doing today, Jeff?
Jeffrey Shaffer:
I'm great, great to be here again.
Adam:
I really appreciate you coming on. I know we kind of were crossing paths on something totally different. And I, you know, as we immediately started going down kind of that technology discussion path, I thought it would be a lot of fun to have you come back on and talk to us about all that has really changed from an artificial intelligence and machine learning. For those of you that are watching, if you haven't seen the first episode, we'll link that one below. But I highly suggest that you go and check that out and get yourself a better understanding of what those terms really mean in the application of a business. But Jeff, for anybody who hasn't seen our last episode, can you tell everybody a little bit about yourself and how you got to the seat that you're in today?
Jeffrey Shaffer:
Sure, grew up in financial services, hence, you know, on this podcast, I guess, 27 years at Unifon, who has been around in the space since the very beginning, back in the mid-80s. Did 10 years running operations, 10 years in the role of IT and analytics, as seven years as the chief operating officer. And then I took a position at the University of Cincinnati. I've been and last year I took a full-time position with them and continue my role at Unifund but I stepped down as the chief operating officer from day to day and just went back to kinda managing the IT and analytics groups and so yeah that's what I spend my time doing. By day I'm teaching classes around data analytics. I'm in the business college but in the business analytics department and then you know Unifund doing all sorts of stuff as well so Unifund and RDS.
Adam:
Well, let's talk a little bit about Unifund and RDS for a minute and I'm pretty sure that just about everybody who's watching this podcast should be familiar with both of those organizations, but can you tell us a little bit about what you guys do there?
Jeffrey Shaffer:
Yeah, well, Unifun probably have heard of one of the debt buyers been in the space forever. We buy accounts similar to some of the other debt buyers in the space, work with the top issuing institutions and creditors and whatnot. We formed RDS, which is Recovery Decision Science in 2015, and went out and started helping others with their portfolios. That could be anything from servicing. We've done master servicing for clients. collection servicing, legal servicing, and manage portfolios in that sense, even document management and handling payments and disputes and complaints and all the fun stuff. But we also build products that we sell and that's really where the analytics and the machine learning and the scoring kind of come into play for us because we took scores and analytics that we have built for ourselves over the years and we started selling them in the marketplace through RDS. And so we formed a Lexus Nexus. We sell a number of our scores through the Lexus Nexus risk department including on the last episode I think I talked about our deep learning model on real estate and doing some fun things around that and it's very predictive in the collection space and we sell that score today and we also have a litigation score that we sell through Lexus Nexus as well. So RDS is you know servicing and products and analytics and even some relatively new stuff, newer things that we're coming to market with, to even upstream like a lending score. So
Adam:
Hmm.
Jeffrey Shaffer:
that's hot off the press. Our latest product will be around not defaulted loans, but consumers who are seeking credit. So we have three new lending scores actually.
Adam:
Wow, well, clearly you live at the intersection of technology and financial services, and especially when it comes to some of these newer and more advanced technologies. And that kind of brings me to my big question here today. As I've been going to a lot of conferences over the past couple of weeks, almost every single one of them has been talking about the CFPB and the CFPB's focus on artificial intelligence. And I... I know you're kind of the perfect guy to be asking questions about this particular intersection here, but what are you seeing from the CFPB, or what are you predicting their real focus, or what they're trying to accomplish in their focus on artificial intelligence and machine learning?
Jeffrey Shaffer:
I think it's pretty straightforward. Here's the historical problem in this field has been that machine learning models have been thought of as a black box.
Adam:
Mm-hmm.
Jeffrey Shaffer:
And so you didn't really know under the hood what that is. And so in traditional statistics, you would have something like an explainable model where you could see coefficients, we call them a positive or a negative, it's waiting in a good direction or a bad direction. And so you would know the reason somebody was, higher score or a lower score or what was creating the outcome. And for many, many years until recently, you know, people really had a black box around machine learning models. And so banks for a long time, for example, would steer away from machine learning models because they weren't necessarily explainable in a traditional sense. Here's the thing is that world's kind of changed. I mean, we have gotten more advanced with machine learning, deep learning models. We now have ways of explaining them. So I think really the essence of what the CFPB is really just saying is you can't just say you got a black box and say, yep, I have no idea what it's doing. It's just producing this outcome. It really comes down to, no, you're going to be held accountable for anything in your model that could be a negative impact to a consumer. For example, the one we always test for is disparate impact analysis, right? or whatever, you have to be concerned about, you know, protected classes and what are your scores doing as far as a disparate treatment of those things. And so, a couple of things, I did test a screen sharing here, so I'll just share something real quick. Here's an example of just something that has just changed in the last few years. I mean, in the last year, people have really been spending a lot of time these models. I find it ironic we're on a technical, you know, technology call and the screen sharing doesn't work, right? It's always these kind of episodes, but let me try it again and see if that'll work.
Adam:
I think you bring up some really good points about the disparate impact and you know, are you actually watching right? And do you understand what the outcome is going to be based on the inputs that you're throwing in there?
Jeffrey Shaffer:
Yeah, and so in a traditional model, you throw all those inputs in, you get the coefficients back out, and you know these are your good variables, these are your bad variables, you know the weightings of those variables. And in a machine learning model, say, let's say for example, a neural network, you have all
Adam:
Yeah.
Jeffrey Shaffer:
these little connections of these variables in the neural network, and you don't really know how they're connected together, like which variable is weighted more or less. analysis, you know, we do these shat values and you know, here's an example of that. And so, you know, we can kind of see like the positive negative impact on the scale. You kind of see like, oh, what is it has a high impact or a low impact and it has a high negative impact or it has a high positive impact. And so it gives you visibility into this. And this is just, you know, one of a few tools that we can use now that, you know, you can well, percent, this isn't my model, this is something from the internet, but tax rate or crime rate or percent of working class. Well, percent of working class is a big value. If it's a lower value, it's a big negative impact, and if it's a higher value, it's somewhat of a higher impact. But you see on the left side, it's got this really, this dark red, right, on the negative sign of working class. You know that this is a high, in your model and so now you know that. And then you know the second thing the CFPB themselves have published disparate impact code so you take that code and you know you're not using any of those variables in your model I hope you're not whether somebody is you know a race or something like that but you know then you can take that race variable and you can proxy it with the CFPB code and you can run disparate impact analysis against your model and here's what the population looks like for my distribution and here's what my model looks like for my distribution and you can kind of compare and say oh my model is not you know it's over lending you know it's lending to if you're a white male and it's not lending to a black male well you could figure that out in your model right and you could know hey there really is disparate treatment of this in some form and so you can go back and you know we don't you'd never use a variable like race but you might use a variable like zip code that
Adam:
Yeah.
Jeffrey Shaffer:
good proxy race and all of a sudden you have this variable in there that you know would proxy something that you didn't intend to do and so this type of analysis kind of helps you figure that out right that you're treating consumers fairly and equitably and in a in a consistent manner and not skewing the model one way or another in treatment.
Adam:
Well, the unintended consequence of the inputs, right? So like you say, being able to load zip code may end up with a result that you weren't expecting.
Jeffrey Shaffer:
Yeah.
Adam:
But it is good that there's some testing that we're able to do at that level. But that kind of brings me to these large language models, right? And so the last time that we talked, we talked about, you know, machine learning. We talked about. deep learning and now we're starting to talk about these large language models. Can you help me understand like where that falls into the spectrum of artificial intelligence?
Jeffrey Shaffer:
Yeah, well, that's the hot new topic, right? So last
Adam:
Definitely is.
Jeffrey Shaffer:
December, OpenAI released their sort of new interface on chat GPT. It's been around for a while, by the way. They had this background sort of interface around for a few years and different models, and nobody really paid any attention to them. And two things happened. Their models got bigger and better. And number two, they put a shiny interface on top of it. And the world took notice. like, oh, this is really amazing. And it's doing some pretty incredible stuff. And then a few months later, they released the bigger version, GPT-4. And all of a sudden, that thing is coding for you in Python and R.
Adam:
Yeah.
Jeffrey Shaffer:
And it's writing documents. And it can answer questions. And it's now these language models have passed the bar exam and passed the medical exam and is in the top tier of SAT scores, right? It's like the output of these things have gotten really incredible. over time and it's moving at the speed of light. I mean, innovation after innovation after innovation. The last time we talked, half the things that exist today didn't even exist. They didn't have these tools available. So it's moving really, really fast. I guess a couple things to note would be. don't get tied up in the term language, large language model, right?
Adam:
Okay.
Jeffrey Shaffer:
It is good at language. It's great at language. That's what its original use case is. But people have figured out, you know, mathematically behind the scenes, it just takes this language and it converts it into numbers in a matrix. It's like a vector. And so you have this vector of numbers. Well, you can take an image and make a vector of numbers. You can take a sound file and make a vector of numbers. You can take a picture and make a vector. So, you know, whether it's video, or images or sound that has now transpired to you know you can take my voice sample my voice with a few you know with just this 15 or 20 minutes we're talking and punch that into a model and put a script to it and it sounds like me sounds like Morgan Friedman or it sounds like Mike Tyson or whatever you know it can learn that video it can it can now create started with images and creating images of anything we can imagine now we can do Whereas the traditional large language models, there's just so many tasks that it's really, really good at. Sentiment analysis, positive and negative, you know, are your customers happy about your product or not. Translation, it's very good at translation. So you want to take something in English and translate it to French or German. It does that really, really well. It does segmentation and classification of things. So you could take statement data from, you know, this is an application. that we've been working on at Unifun, it'll take ExxonMobil or it'll take Walmart or WM Super Center and it'll classify that as fast food or gas or department stores, things like that. So there's so many applications of what it can do. Personally, I think the biggest, in my world, the biggest applications are data analysis. It's gonna change the way we do EDA, exploratory data analysis, it's going to just change the way that we do that, and coding. You know, you can, you don't have to be a coder anymore. You can just ask chat GPT to write you a code and it'll spit that code out. And again, I mean, since I can share my screen, I'll just, you know, give you a little demonstration here if it comes up. This is Chat GPT here. So I have the plus version. I pay a subscription to this. Up here, there's advanced data analysis that's in beta, but it's live and working. But if I just use the default model, I'll just say list the first 10 presidents of the US. And it'll tell me the first 10 presidents of the US. It gives me dates of when they were in office and so on. While it's doing that, I can say, hey, a Python script to count the... words in a CSV document. So how many words are in my document and I'm asking it to write a Python script. So here goes the Python script. It's going to say, yeah, we can do that. It's going to give me the code. It's going to pop up a code window here. There's my code for Python. I don't have to code anything. I can just run that code in my Python window and it'll figure out how to count the words in a CSV file and do all that stuff. So that's a good example. I mean, we could go in here and click on this advanced data analysis. Check this out. I'm going to open up, I'll just open up a file here. I'll just go to my data window and I'll just pick the Titanic data for fun. And I'll just say, tell me about this, right? I just dumped a CSV file in here. I didn't even, it has the name Titanic in it, but I didn't say anything about it. Well, what it's doing right now is it's writing Python code to analyze the file. It's going to look at its analysis and it's going to start telling me about this file How many people survived how many passengers are there the sex it has to tell me all the fields and then I can just say Do some EDA on this file right EDA is Exploratory data analysis. I didn't I'm not going to tell it what it is It should know what it is, but do some EDA on this file Certainly some exploratory data analysis, let's look around and it's going to go run some basic statistics and look at missing values and it'll look at the distribution and the correlation, it'll create some visualizations. So now it's writing its own code, it's running its own code, it's giving me the results of the code. I mean imagine being able to do this on your data at your office, like do you need a data analysis person, right? Like you can do some of this yourself. is going to be looking at data, it's telling me, oh, by the way, the mean of this, or the age of this, or the ranges of the passenger ID, now it's checking for missing values, and it's telling me there's 687 missing values in cabin, and now it's running a data distribution. So this, to me, is the future of where everything's gonna go.
Adam:
I think you're, I mean, wow, I'm a little floored at the level of tool set. How does the tool set do with the visualization of data? I know that's kind of something that like you've always really lived in.
Jeffrey Shaffer:
Yeah, from an exploratory standpoint, it does it really well because it will, it says right here, data distribution, let's visualize the distribution, would you like me to proceed? So now it's writing code to create visualizations. I think from an exploratory standpoint, it's already there. From a polished present to an executive in a slide deck, nah, it's not there yet. You know, you would probably have to take this code and, you know, you do something with it, right? But
Adam:
Mm-hmm.
Jeffrey Shaffer:
look at that. I mean, it just popped out the distribution of these things. It's giving me it's giving me the fair, it's giving me the P-class, it's you know whether they survived or not, and then you know it's telling me things about it right? Like the distribution of the age and here's the fair distribution and here's the sex, there were more males than females on board, and so now it wants to go into analysis, it wants to correlate variables together. I'll say yes, go ahead and proceed, and so now it'll do analysis, and by the way it'll even write whether a passenger is going to live or die. And so that's interesting. I think this will be for all of us in the industry and other industries, this is kind of the future of where that stuff is going.
Adam:
So you see this is kind of like the future of stratification and many aspects as well, right? Cause if you're trying to understand what's within a large data set, I mean, this is the capability of visualizing that information and looking at an anal, like analyzing it in a number of different ways. I mean, it's almost an infinite breakdown.
Jeffrey Shaffer:
Yeah, but
Adam:
Where do you start hitting the
Jeffrey Shaffer:
it
Adam:
Peter
Jeffrey Shaffer:
ties
Adam:
principle?
Jeffrey Shaffer:
back, if I can jump in, it ties back to your first question about the CFPB because now you say, okay, well, you need to explain your model. Look at this. It just ran a data set that we didn't do anything with and it says what the data is doing, right? There's a negative correlation between this and that. There's a weak correlation between this and this. There's a positive correlation between survived and fair, and there's a strong negative correlation between P-class and fair. So I mean, talk about explainable model. all the interactions of all the variables with me on the fly.
Adam:
That is wow. I mean, that is exactly what the future, I think is starting to look like. Now, I know when it comes to a chat GDP, one of the things that we are constantly hearing about is like what happens when you're feeding the model and are you feeding that model, proprietary information versus non-proprietary information. How does that break down in terms of the application for a financial services firm?
Jeffrey Shaffer:
That's a great question. I didn't even feed that question to you, but you know what? That is an awesome question.
Adam:
I'm...
Jeffrey Shaffer:
The future of AI, I think, is gonna be open models that people develop themselves. There's a website called
Adam:
Yeah.
Jeffrey Shaffer:
Hugging Face, which is sort of like the GitHub of the machine learning world, and it has all of the open LLMs. And so these are all open source, commercially viable models that people can download and use, and some require heavier equipment than others. these 70 billion parameter models, these are at the top of the scoring list. So these are the best performing models. But just to give you an idea, this Lama 2 model has been at the top of the list for the last six weeks or so. A lot of Lama 2 on here. And it's been scoring really, really well. And it's comparable to CHAT GPT and it's an open source model.
Adam:
Hmm.
Jeffrey Shaffer:
I can in fact... I am on a web NR. I have my video running, I have, I don't know how many tabs, 100 Chrome tabs running,
Adam:
I'm
Jeffrey Shaffer:
I have
Adam:
sorry.
Jeffrey Shaffer:
Tableau open, I have Notepad open, I have all this stuff open on my machine at this moment right now. This is an interface to the Lama 2 model that is running on my laptop. I could literally disconnect from the internet right now and this model would run. And I asked it before to write a short paragraph here. I'll ask it the same question I asked. previously which is you know list the first 10 presidents of the US. Now imagine you're at a bank you can load this model up on a server you can run this on a server it's running just like chat GPT your data is not going anywhere it's in a secure environment yet it's acting just like chat GPT write a Python script on spell it correctly to count though in a CSV file and now I have it. This is a 13 billion parameter model. It takes up about 10 gigs on my laptop. I have a high end video card on my laptop like a gaming computer so it can
Adam:
Mm-hmm.
Jeffrey Shaffer:
run. But look at this. It's generating Python code. It's answering questions. It's doing all of this stuff that ChatGPT is doing and I don't even have to be connected to the internet. It's fully siloed, right? So the future of this is you're going to take a Lama which is running over here, this is where I load my model. I'm gonna have a Llama 2 model, and I'm gonna take Llama 2 and take my medical data and attach it to it. I'm gonna have a medical database. I'm gonna take my financial data and tie it to it. I got a finance database. So I'm gonna build custom proprietary coding based on one of these language models. I'll basically fine tune it and create more embeddings from my own data. Imagine every law firm having access to all the cases. They'll have,
Adam:
It's
Jeffrey Shaffer:
you know, legal llama, right? It'll be legal llama model. So yeah, that's I think people will not put their data on chat GPT. They'll they'll run it locally.
Adam:
I'll be able to run all that locally. I, and it, it's interesting when you talk about the, uh, the physical requirements to run that kind of, um, that kind of model, being able to run that from any kind of a laptop is just mind blowing. Um, I mean, it's really mind blowing. I think that it doesn't
Jeffrey Shaffer:
Yeah,
Adam:
even
Jeffrey Shaffer:
no,
Adam:
require
Jeffrey Shaffer:
I mean...
Adam:
you to load that to the cloud.
Jeffrey Shaffer:
At our office we have a server that has a Unifun, we have a server that has some high end video cards in it that we use for deep learning. We could run it on there, you could get a high end video card, they're now getting very expensive. I mean you need a $5,000, $6,000 video card at the start to do some of this stuff and that can go up to $30,000, $40,000, $50,000 to get a video card to do this. But that's where I think the big companies are kind of going to go to. you know versions of this based on their own proprietary data.
Adam:
No, I mean, that's the best explanation I've ever heard about how we'd be able to actually leverage the tool set without having to commingle data, right? Commingling data from a whole bunch of different places and different ownership and all the things that come along with that. Wow. I, Jeff, honestly, I kind of blew my mind a little bit again today. I really appreciate you coming on and having this chat with me. I know. I just learned a lot from this conversation about the application of how these technologies and tools might actually be usable. And although I've seen a whole bunch of presentations on it lately, I haven't had anybody who's actually been able to, they've just posed the questions. This is the first time where I'm really hearing the answers to the questions about how do you keep that information separated and segregated? How are we actually going to be able to use these models? How can you address the black box scenario that the CFPB sees? And you're literally talking about the model explaining the model itself. So I'm just, at this point I feel like a student in one of your classes or like I need to be going to the University of Cincinnati here just to continue to learn on this. Wow, I just, I can't thank you enough for your time today. This is amazing.
Jeffrey Shaffer:
It's always a lot of fun and if you're interested in learning more about any of our prediction models that we've developed at RDS, like I said, we have litigation prediction if you're doing lawsuits, you know, are you suing the right people? You know, we think we can probably help you there. The real estate score is very predictive in many aspects of what we're doing and so, yeah, we have a suite of products and if you don't want to do it yourself, you can hire us as a servicer too. So yeah, check out recoverydecisionscience.com.
Adam:
Well, I will put links to both Unifund and RDS below to make sure that anybody who's interested in learning more can easily find you guys, because I think you're doing some really amazing things in an industry that's so heavily regulated for you to be able to find the avenues that enable you to execute on these things while staying within the parameters and guardrails that have been set up is just truly amazing. For those of you that are watching. If you have additional questions you'd like to ask Jeff or myself or additional topics you'd like to see us cover, you can leave those in the comments on LinkedIn and YouTube. And hopefully I'll be able to get Jeff to come back again and continue teaching me about AI, machine learning, large language models, chat, CDP. I mean, what a great conversation. I just can't thank you enough for this one, Jeff.
Jeffrey Shaffer:
I'd be happy to, yeah. Thanks for having me.
Adam:
Absolutely. And for those of you who are watching, again, if you have any questions, comments, leave them below and we'll talk to you all again soon. Thank you so much, everybody.
Jeffrey Shaffer:
Thanks.